There’s a blog post that floats around the Internet about the metaphor of the broken stair. In this blog post, the author talks about how certain social groups have a person who before you talk with them, someone will pull you aside and say, “Hey, listen, be careful around John. He can be touchy, so you probably shouldn’t let yourself be alone around him.”
The author of the blog post points out that this is a lot like people who pull you aside right before you go down the stairs to the basement and say, “Hey, be careful on the third stair. It’s broken, and if you step on it, you’ll go right through to the concrete floor.” Somehow, these people find it easier and more logical to warn every single person before they go down the stairs than to just fix the broken stair (which, in the case of John, would probably involve just banning him from parties). As the author of the blog post points out, this is pretty illogical. We should just fix the problem.
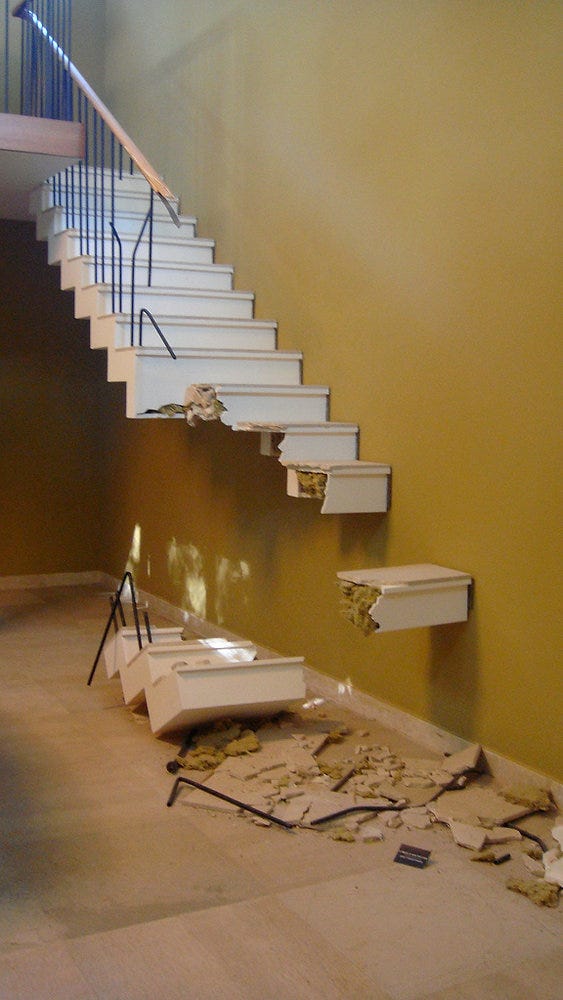
But, unfortunately, there’s a big gap between “should” and “will”. Fixing a broken stair well enough that people can reliably stand on it takes money, time, and effort. Warning people about it takes only a tiny bit of time and effort. And, to be cynical about it, forgetting to warn someone about the broken stair is going to get people a lot less mad than trying and failing to fix the stairs. It’s easier just to let it lie, but, ultimately, worse for everyone.
I was thinking about this blog post recently as I once again dug into the mysteries of pharmacokinetics while traveling up my own drug development staircase. The drug development staircase is always rickety and some steps will never be that sturdy, but the pharmacokinetic stair seems particularly broken, and it frustrates me that it is.
Before I start complaining about it though, I should probably explain what pharmacokinetics is. Pharmacokinetics is the study of everything that happens to a drug when you put it in your body. So, if you’ve ever asked questions like “Why does my Advil take a few hours to work?” or “Why do I have to take a Claritin every 12 hours?” or even “Why does asparagus make my pee smell funny?”, well, those are all pharmacokinetic questions.
If you look on the Wikipedia page for pharmacokinetics, they make it seem very straightforward. They explain that the traditional acronym for understanding pharmacokinetics is LADME: liberation, absorption, distribution, metabolism, excretion 1. So, you ingest a drug; the active ingredient is “liberated” from the capsule or however it’s been contained; the active ingredient is absorbed into your bloodstream; your bloodstream distributes it around your body until it eventually ends up at your liver/kidneys; your liver metabolizes it; and then you excrete it in either solid or liquid form.
Like all good acronyms, LADME transforms the amorphous into the straightforward. You no longer have to ask “how does oral amoxicillin know to go to my infected toenail” or “why does my Claritin wear off after a while”. Amoxicillin is simply distributed around the body by your bloodstream and, at some point, it will reach your toe. Claritin lasts as it does because, at some point, it gets metabolized and then excreted out, at which point it’s no longer effective.
Thinking about things in terms of LADME also allows us to start diving deeper into individual topics, and makes them quantifiable. So, distribution and metabolism becomes Cmax, the maximum blood concentration that a drug reaches. Or, metabolism and excretion become t1/2, the amount of time it takes for the concentration of the drug in the bloodstream to reach half its maximum value.
None of this stuff is wrong and all of it is useful. I’ve used it myself in thinking about my company’s lead drug and how often and what level we should dose it. But, as I alluded to before, there are some real issues that pop up if you start digging into it.
The first big problem is that the numbers that we get when we do the math for Cmax, or t1/2, or AUCinf 2 are all descriptive, not predictive. We get these numbers by giving a bunch of people (or, if you’re Highway Pharmaceuticals, cats) some dose of the drug. We try to match these people up and make sure they’re all roughly the same weight, have eaten roughly the same food, aren’t on any drugs, and don’t have any health problems that would impact their organs. Then we measure the concentration of the drug in their bloodstream over a set series of timepoints, and do statistics from there.
This definitely provides us with some knowledge. But it’s also a lot like understanding physics by shooting a thousand ping pong balls from a cannon and measuring their height at a bunch of different time points. Yes, that would be somewhat informative. You could say stuff like, “Here’s the maximum height that an average ping pong ball reaches” or “Here’s the average distance that a ping pong ball shot from a cannon travels”. You’d be able to predict pretty well what would happen to the next ping pong ball that was shot from a cannon, and have a rough idea of what would happen to a watermelon.
But it’d be a stretch to try to go from there to figure out what would happen to a bullet shot from a pistol, and it’d be impossible to figure out what would happen to an asteroid slingshotted by a planet. These aren’t just simple extrapolations from existing data. In order to make these predictions, we have to understand the underlying processes.
To drug developers and pharmacologists, this isn’t surprising. They already know this. This is why they embark on their expensive pharmacokinetic trials in animals and humans when they develop a drug. It costs a lot of money to do these, nobody knows what’s going to happen before they run the trial, and bad results can sink a drug or at least take it back to the design phase 3 4. Companies and the FDA try to avoid drugs with bad pharmacokinetics, which are not just drugs with really short half-lives, but often just drugs with unpredictable pharmacokinetics, like a cannon that sometimes shoots ping pong balls 1 foot and other times 100 feet.
And, for drugs with unavoidably bad/unpredictable pharmacokinetics, like cyclosporine (nb: the bad pharmacokinetics of cyclosporine are exactly what my startup is trying to fix), there’s a whole subfield of medicine called therapeutic dose monitoring, which is devoted to how much different drugs’ pharmacokinetics need to be monitored, how best to do it, and how to use predictive statistics to extrapolate from the fewest number of time points.
But this feels less like addressing the problem and more like just avoiding it or working around it. It’s like the classic broken staircase metaphor: if you start drug development, everyone will warn you about pharmacokinetics, and then it’s up to you to figure out your way around it with the right combination of consultants, drug design, etc. to bridge the gap.
It just seems crazy, though, that nobody is putting serious money into actually putting a stair into that gap. I mean yes, there is a small “physiologically based pharmacokinetic modeling” community, which tries to model the whole LADME process as a bunch of differential equations, but they are, to put it politely, struggling. Their differential equations are on shaky ground, especially as they don’t even have human values for a lot of their diffusion constants. They have to rely on rat values from old papers for information on how, say, molecules are transported across liver membranes. This is very far away from being able to capture even the mean human pharmacokinetic experience, nevermind the diversity of actual experiences.
We could fix this stair. It would just require the raw data from a variety of pharmacokinetic trials, some in-depth experiments on human liver and gastric membranes, and some simulation of the physics of how different drugs diffuse into the bloodstream and across membranes. This would be difficult, but not impossible, and would not require any huge scientific advances. If it were done, it would likely save hundreds of millions, if not billions of pharma dollars each year, improve or even save the lives of the thousands of people who depend on therapeutic dose monitoring (e.g. every organ transplant recipient), and get us way closer to obviating healthy human trials altogether.
But, you know, it would require coordination and money. So, for now, we’re just going to keep on watching out for that stair, and woe unto anyone who plunges through. Hope you have health insurance!
Sometimes, people put toxicology at the end to make LADMET, but I dislike this. Toxicology is a different discipline and you don’t really test it with pharmacokinetic trials.
Also, really traditional people don’t include the liberation step, as they consider that to be drug design. I don’t feel strongly about this choice.
Area under the curve as it goes to infinity, which is generally understood as the total exposure someone gets from a given dose of drug.
Take GLP-1 agonists, the miracle weight loss drug class du jour. Semaglutide (a.k.a. Ozempic or Wegovy), which is currently selling so well that it is making Denmark’s Central Bank struggle to rebalance the krona, was not the first GLP-1 agonist. In fact, we’ve known how to literally make GLP-1 for a long time.
But you can’t just give GLP-1 to people. It has terrible pharmacokinetics. It’s not available orally, and intravenously it has a half-life of 2 minutes. People would have to be injecting themselves non-stop to see any weight loss.
Semaglutide is successful because it is a GLP-1 agonist with good pharmacokinetics. Novo Nordisk spent billions making sure this was true, including multiple expensive pharmacokinetic trials. It took about 20 years. It would have been nice if they could have avoided some of those trials!
Assuming you’ve read the previous footnote, you’ll know that the big innovation of semaglutide was improving the pharmacokinetics of GLP-1 agonists. If you’re curious, here’s what they did. I’m basing this account off of my limited understanding of the account of the inventors themselves.
The central problem with using biologic GLP-1 as a therapy, as mentioned, was that it had a short half-life. So all efforts were focused on creating a version of GLP-1 that had a long half-life.
The old methods of creating a drug with a long half-life don’t work for a giant peptide like GLP-1. It’s way too big and it’s a fatty acid. So, a new way of creating a drug with a long half-life is to bind it to albumin, which is an all-purpose carrier protein in the body. Albumin is naturally really good at carrying things: it has several binding sites, a long half-life, and the body allows it to exist in high concentrations in plasma.
In order to get albumin to carry a peptide, though, you need a peptide-based ligand, or something to chemically attach the protein to the albumin. Before any of the stuff with semaglutide, a lot of work had been done to try to find these ligands. The original team built off this work to find ligands that could reversibly bind to albumin (i.e. GLP-1 could be attached to albumin for transport, and then unattached for use).
In order to see what they could attach to GLP-1 without messing up its function, they employed an alanine scan. In an alanine scan, each amino acid in a peptide is subsequently replaced by alanine to see which amino acid actually does. It’s sort of like figuring out how a radio works by taking one piece out at a time then seeing how the radio functions without it.
From there, they found the parts of GLP-1 that were necessary for its function and shouldn’t be messed with, and then the parts that weren’t necessary and could have ligands attached to it. Then, they again used the technique of creating a bunch of versions of GLP-1 with ligands attached to it to see which had both a good attachment to albumin and were still functional.
From this exploration, they created liraglutide, the first effective GLP-1 agonist, which you might have heard of as Saxenda or Victoza. This drug works as a GLP-1 agonist (which is why it’s approved), but it doesn’t work that well.
Novo Nordisk took this work and improved on it by noticing that compounds that bound really well to albumin bound less well to GLP-1 receptors, probably because there was competition between them. So, they wanted to create a sequel to liraglutide that still lasted a long time, but was a better agonist for GLP-1 receptors.
So they modified liraglutide to not only have better affinity for GLP-1, but also to have an additional piece to connect to albumin that would keep the GLP-1 parts of liraglutide away from the albumin parts. A good analogy might be to think of old liraglutide as having a plug for GLP-1 receptors next to a plug for albumin, which made it so it was tricky for liraglutide to simultaneously plug into its carrier, albumin, and its target, GLP-1 receptors.
Semaglutide has two new plugs that are not only better fits for only their intended targets (i.e. the GLP-1 receptor plug fits better into GLP-1 receptors and worse into albumin and vice versa for the albumin plug), but they also are far apart from each other, so they are less likely to physically interfere with each other.
All of this stuff is way harder than it sounds, because semaglutide and liraglutide are large fatty acids that can’t be crystallized. I think the best analogy would be designing a magnetic pool toy that’s designed to connect to two other separate, magnetic pool toys simultaneously, but all of the pool toys are transparent and slippery, and the only way you have of connecting them is by stirring a bathtub with a spoon. You can see how questions like, “How do I make these pool toys connect better?” or even “How can I tell if they’re connecting?” get really, really difficult.
> All of this stuff is way harder than it sounds, because semaglutide and liraglutide are large fatty acids that can’t be crystallized. I think the best analogy would be designing a magnetic pool toy that’s designed to connect to two other separate, magnetic pool toys simultaneously, but all of the pool toys are transparent and slippery, and the only way you have of connecting them is by stirring a bathtub with a spoon. You can see how questions like, “How do I make these pool toys connect better?” or even “How can I tell if they’re connecting?” get really, really difficult.
Loved this, and excellent essay.
Why do some people respond to certain drugs but not others? Frequently the answer is “nobody knows, let’s try a different treatment.” I suspect this usually means there’s at least one hidden variable or mechanism nobody has discovered yet, let alone measured…
In your ping pong ball example: imagine we recorded a bunch of height/distance data but without being aware of concepts like drag, temperature, barometric pressure, wind speed and direction, the coriolis effect, etc. My layman’s intuition is that this is our current state of pharmacokinetics — we don’t have a complete model but we also don’t even know what data we need to build one, and this a complicated system with hundreds or thousands of things going on and millions of variables. Which ones are important? It’s hard to say what approach would be best for tackling this